Teaching Robots to Do Chores: MIT’s RialTo Revolution
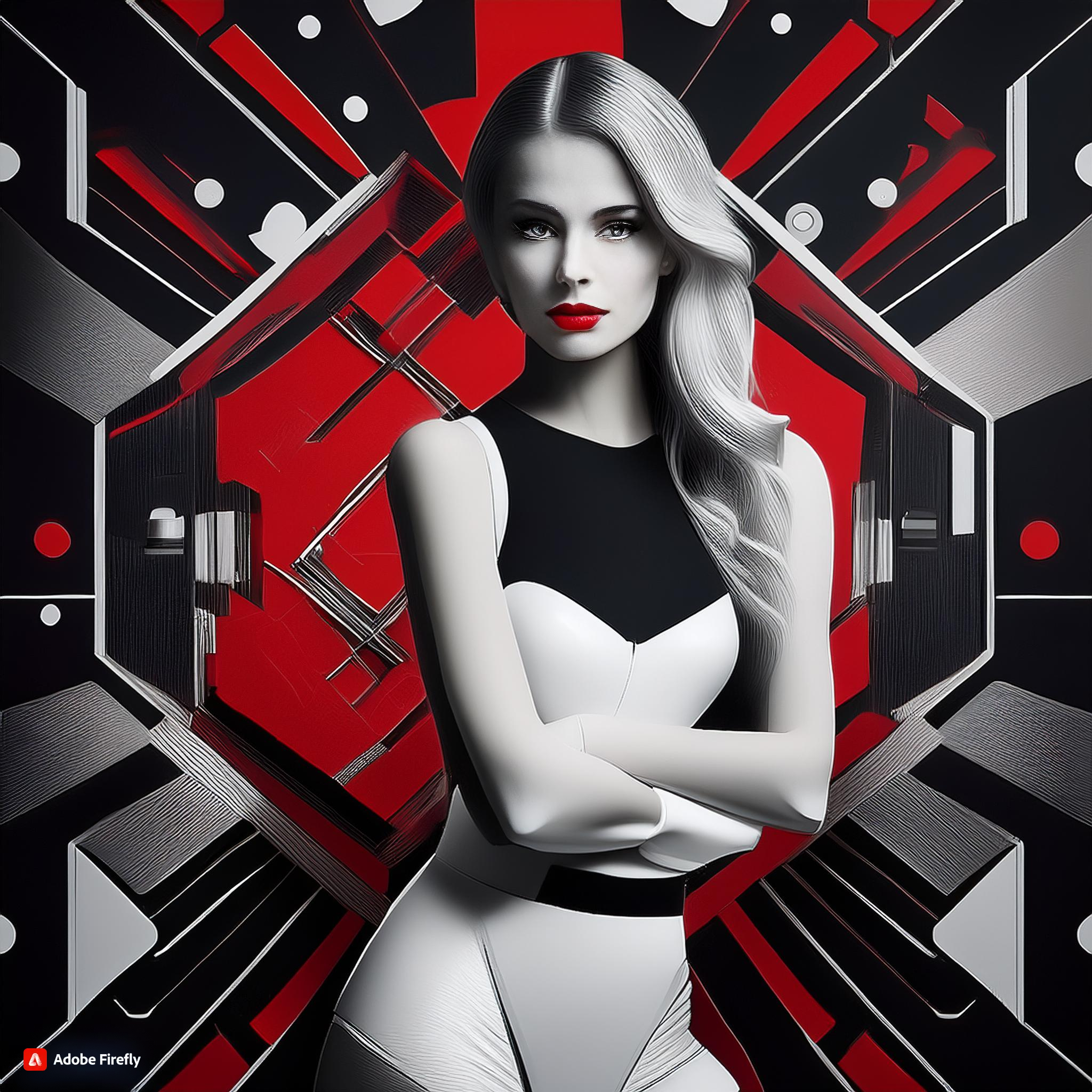
To achieve widespread adaptability, robots must learn generalist policies. MIT CSAIL's Real-to-Sim-to-Real model, RialTo, tackles this by creating digital twins of real-world environments. Users can capture their surroundings with a phone and upload the data to RialTo, which then simulates these environments to train robots. This process, leveraging GPU parallelization, enhances policy learning by 67% over traditional imitation learning. Key tasks tested include opening toasters and drawers, placing books and mugs on shelves, and more.
Why It Matters:
- Improved Training Efficiency: Reduces reliance on extensive reward engineering.
- Broader Applicability: Prepares robots for diverse real-world scenarios.
- Enhanced Robustness: Ensures performance under disturbances and distractions.
In practical tests, RialTo outperformed traditional methods, especially under challenging conditions like random object poses and physical disturbances. By utilizing reinforcement learning in simulations, robots developed strong policies transferable to real-world applications. Zoey Chen, a Ph.D. student at the University of Washington, praised RialTo for addressing safety and efficiency constraints, making it a significant advancement in robotics training.
Despite its promising results, RialTo faces limitations. Training currently takes three days, and simulating complex elements like deformable objects remains challenging. The team plans to enhance the system with foundation models to speed up training and improve adaptability. Continued efforts aim to minimize human input and achieve broader generalization capabilities for robots.
Key Highlights:
- Tasks: Opening toasters, placing books and mugs on shelves.
- Improvements: 67% policy enhancement over imitation learning.
- Testing: Performance under varying difficulties and real-world disturbances.
Reference:
https://www.therobotreport.com/mit-csail-teaches-robots-to-do-chores-using-real-to-sim-to-real/