Can Robots Dream: MIT’s Research on “Machine Dreams”
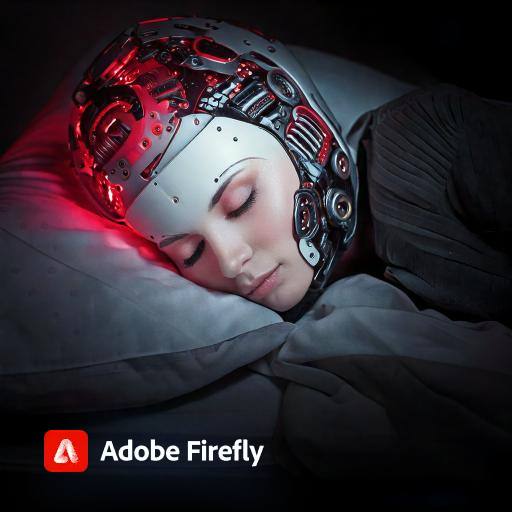
A New Approach to Robot Learning
In November 2024, MIT researchers introduced a groundbreaking concept that could transform the field of artificial intelligence: "machine dreams." This method seeks to expand how Intelligent Agents acquire skills and solve complex problems. Unlike traditional training, which depends solely on real-world or simulated data, this approach allows robots to create their own "dreams" to explore scenarios and enhance learning.
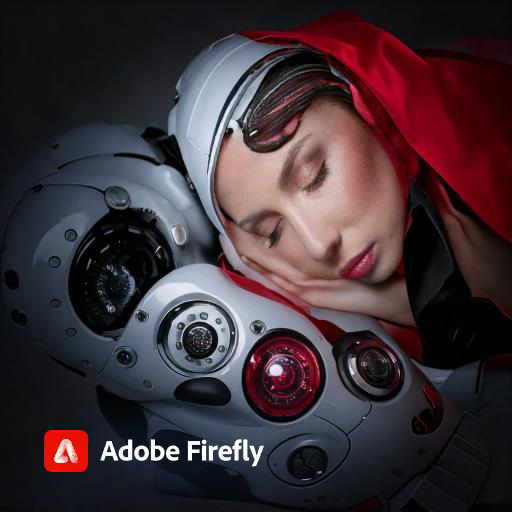
How "Machine Dreams" Work
The concept involves enabling robots to generate internal simulations and use them to refine their learning processes. These machine dreams let robots perform various tasks, test outcomes, and optimize strategies without outside data. This approach is significant because it can reduce the time and resources needed to train complex AI systems. If proven effective, it could result in robots that are more adaptive and capable of performing tasks with minimal supervision.
Potential Applications and Examples
Experimental data from MIT shows that machine dreaming can improve the decision-making accuracy of AI systems. This breakthrough could be highly beneficial for fields like healthcare and manufacturing, where adaptability and fast learning are crucial. For instance, Non-Human Workers that learn independently through internal simulations could enhance productivity and precision in these critical sectors.
Impact on Autonomous Systems Development
This advancement in robot autonomy highlights progress in artificial intelligence and raises questions about the boundaries between human-like cognitive processes and machine capabilities. As technology advances, "machine dreams" may become a fundamental aspect of autonomous systems, shaping the future of Digital Employees and their role in industry and daily life.
Key Highlights:
- New Concept Introduced: In November 2024, MIT researchers explored "machine dreams" as a new method for robot learning.
- How It Works: Robots generate internal simulations to practice tasks, test outcomes, and optimize their learning without external data.
- Benefits: This approach can reduce training time and resource use, making AI systems more adaptive and capable of handling tasks independently.
- Evidence of Success: Experimental data indicates improved decision-making accuracy when AI systems use simulated dreaming.
- Potential Applications: Useful in healthcare, manufacturing, and other fields requiring quick adaptability; could enhance the role of Non-Human Workers.
- Significance: Raises questions about the parallels between human cognitive processes and machine learning, potentially reshaping the development of autonomous systems.
- Relevance: This method could become central to future advancements in intelligent robotics and Digital Employees.
Reference:
https://news.mit.edu/2024/can-robots-learn-machine-dreams-1119