Top Open-Source Speech Recognition Models and AI Voice Tools
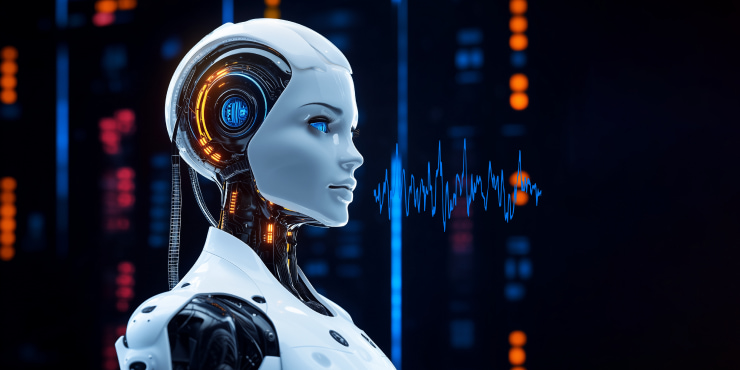
There are many useful software applications based on technologies. Among them, speech-to-text is widely used. We will look at some of the best options for 2024. The technology works by analyzing sound waves and converting them into text using algorithms. Speech recognition software improves productivity, accessibility, and hands-free operation. It allows users to create text materials quickly and efficiently. The choice of software depends on user requirements. At the heart of this innovation lies the ASR model (Automatic Speech Recognition). This system converts spoken language into text with high accuracy.
Open-source tools are vital in advancing speech recognition technology. They provide developers and businesses with free or low-cost access to powerful resources. The growing popularity of open-source platforms demonstrates their potential to democratize AI. They are like Whisper, Kaldi, and DeepSpeech. These models not only support diverse languages. It also offers robust performance for individual developers and enterprises.
What Are Open-Source Speech Recognition Models?
ASR systems convert spoken language into text using advanced algorithms. They power a range of applications ranging from virtual assistants to transcription tools. Open-source ASR models differ from proprietary solutions. The distinctions lie in their accessibility, flexibility, and community-driven nature. They offer unique advantages for developers and businesses.
Key features of open-source models include:
- Differences from Proprietary Solutions: These tools allow users to change the code. Proprietary systems keep their algorithms hidden. Open-source models can be easily integrated into diverse projects without licensing restrictions.
- Definition and Functionality: Speech recognition models analyze and process audio inputs. They generate accurate text outputs. Open-source models provide access to source code. They enable users to adapt them for specific requirements.
- Benefits of Open-Source Models: Developers can tailor models to handle unique languages. They may manage accents, or domain-specific terminology. Open-source tools eliminate the need for expensive licensing fees. They make them accessible for small businesses and startups. An active user base contributes to updates, troubleshooting, and feature enhancements. It ensures continuous improvement.
Developers must leverage text to speech open-source recognition models. They gain the freedom to innovate and maintain control over their solutions. It makes these tools indispensable in modern AI applications.
Key Features of Effective Open-Source Models
Open-source models are essential tools for developers and businesses. Their effectiveness depends on several critical criteria. They ensure reliable performance and ease of use. Let’s explore these features.
There are key criteria for effective open-source models:
- Accuracy: High-precision transcription capabilities are a must for converting spoken words into text. Effective models adapt to varying accents, noise levels, and speech patterns.
- Language Support: Comprehensive support for multiple languages increases accessibility. Advanced models often handle regional dialects and multilingual inputs.
- Easy Integration: Open-source AI voice tools must integrate with existing applications or workflows. Lightweight APIs and modular designs enhance adaptability.
Let’s discuss the role of machine learning and datasets:
- Machine Learning: Continuous improvement relies on AI-powered algorithms. They are capable of adapting to new data.
- Datasets: Quality and diversity of training datasets directly influence model performance. They ensure accuracy across different use cases.
Open-source speech to text models combine precision and robust learning capabilities. They empower developers to create powerful and versatile voice-to-text solutions.
Challenges of Open-Source Speech Recognition
AI voice models offer flexibility and cost-effectiveness. They come with challenges that developers and businesses must address. Understanding these hurdles is important to maximize the benefits of speech to text AI open source tools. Here are common challenges:
- High Resource Requirements: Open-source models often require substantial computing power. This is crucial for training and fine-tuning. This can lead to increased costs for hardware and energy consumption.
- Lack of Professional Support: Open-source solutions typically lack dedicated customer support. This is unlike proprietary tools. Users must rely on community forums or personal expertise to resolve issues.
- Complex Implementation: Setting up and customizing these models can be technically demanding. Developers with limited experience may face a steep learning curve.
Let’s explore the ways to mitigate challenges:
- Optimize Resources: Use cloud-based platforms or pre-trained models to reduce computational demands.
- Leverage Community Support: Engage with active user forums for troubleshooting and best practices.
- Invest in Expertise: Collaborate with experienced developers to ensure efficient setup and integration.
Businesses must address these challenges. They can harness the full potential of AI voice open source tools. Businesses will also minimize risks.
Top Open-Source Speech Recognition Models
The best open source models provide great solutions for developers. There’s a comparison of popular models. These are Whisper, Kaldi, and DeepSpeech. It highlights their features, strengths, and use cases. Check the table below:
Model | Key Features | Strength | Use Cases |
Whisper | Multilingual support, training on extensive datasets. | Exceptional at handling diverse accents and languages. Reliable accuracy across varying speech patterns. | Transcribing global content and multilingual conversations. Ideal for media transcription and accessibility tools. |
Kaldi | Highly customizable modular framework. Advanced tools for acoustic and language model training. | Adaptable to different industries and research contexts. Strong community support for continuous innovation. | Speech recognition in academic and enterprise projects. Tailored solutions for unique datasets. |
DeepSpeech | Lightweight architecture based on deep learning. Focused on real-time processing. | Simple API for easy integration. Efficient for developers seeking minimal setup. | Embedding speech recognition into applications and devices. Projects requiring fast deployment and real-time voice processing. |
Let's compare Whisper vs. Google Speech-to-Text to help businesses and individuals make the right choice:
- Whisper: It offers robust open-source solutions. They are suitable particularly for multilingual transcription.
- Google Speech-to-Text: This tool excels in enterprise-level integrations. It requires subscription fees.
For those seeking flexibility, Whisper is ideal. Google is better for large-scale, automated workflows. These best open source speech to text models are key. They empower developers and businesses to implement cutting-edge solutions fully tailored to their needs.
AI Voice Tools and Their Role in Speech Recognition
AI voice tools are critical in enhancing speech recognition capabilities. They offer solutions for transcription, virtual assistants, and accessibility. These tools leverage cutting-edge technologies. Among them is an open source audio to text framework. It provides flexible and cost-effective applications. These include:
- Transcription: Convert speech into text with precision. Commonly used in note-taking, meeting documentation, and media captioning.
- Virtual Assistants: Power assistants like Alexa, Siri, and Google Assistant. Enable natural language interaction and contextual understanding.
- Accessibility: Assist individuals with disabilities. It offers voice-controlled navigation and text-to-speech features. Enhance inclusivity by breaking communication barriers.
Here are examples of tools that complement open-source models:
- Whisper Integrated Tools: Ideal for multilingual transcription. Often paired with open source AI voice systems to improve accuracy across languages.
- Kaldi-Enhanced Platforms: Adaptable to specific industries, offering customized audio-to-text solutions. Frequently used in enterprise-level software.
- DeepSpeech Applications: Integrated into lightweight devices and real-time transcription apps. Popular for developers building user-friendly interfaces.
There are advantages of AI voice tools:
- Cost-Effectiveness: Open-source frameworks reduce development costs. They also maintain functionality.
- Customization: Developers can tailor tools to unique needs. They may do it with the help of AI voice open source.
- Community-Driven Improvements: Open-source tools are backed by active communities. They ensure regular updates. Such tools enhance compatibility and features.
What is the future Impact of AI voice tools? Let’s explore:
- Enhanced accuracy with context-aware transcription.
- Broader language support to bridge global communication gaps.
- Seamless integration into smart home systems and business workflows.
Businesses and developers must leverage these tools to create innovative applications. They bridge the gap between voice and technology.
Future of Open-Source Speech Recognition
The future of open-source speech recognition is bright. It is driven by emerging trends and expanding applications across industries. Advancements in AI technologies are making ASR voice recognition more accurate than ever.
Let’s discuss the emerging trends in open-source speech recognition:
- Multilingual Support: Tools like Whisper are advancing in recognizing and transcribing multiple languages. Ideal for global applications, from customer service to content creation.
- Real-Time Processing: Enhanced algorithms enable immediate transcription and translation. Beneficial for live events, virtual meetings, and accessibility tools.
- Improved Contextual Understanding: AI models are evolving to interpret context, tone, and intent. These advancements improve accuracy in dynamic environments.
- Cloud and Edge Computing Integration: Combining open-source models with this ensures efficient processing. It happens on local devices. This reduces reliance on internet connectivity and enhances privacy.
Here's how these tools are used across industries:
- Healthcare: Open source audio to text tools can streamline patient documentation. Enable voice-powered interfaces for electronic health records (EHRs).
- Education: Real-time transcription enhances accessibility for students with hearing impairments. Used for creating subtitles and interactive learning content.
- Business Automation: Integration of ASR recognition in workflows optimizes productivity. It also powers virtual assistants and automated transcription. Automation increases customer service chatbots.
- Accessibility: AI voice open source systems enable seamless voice navigation. It suits individuals with disabilities. Breaks barriers in digital and physical spaces through voice control.
Open-source speech recognition will be transformative. Industries will increasingly embrace automation and personalization. Its scalability and cost-effectiveness ensure broader adoption. Advances in AI-driven tools will redefine human-technology interaction. Open-source innovation will remain a cornerstone of accessible and inclusive AI, ranging from empowering small developers to enabling global applications. Follow Newo.ai blog for more information.