The Edge of AI Conversations: Deep Learning Insights
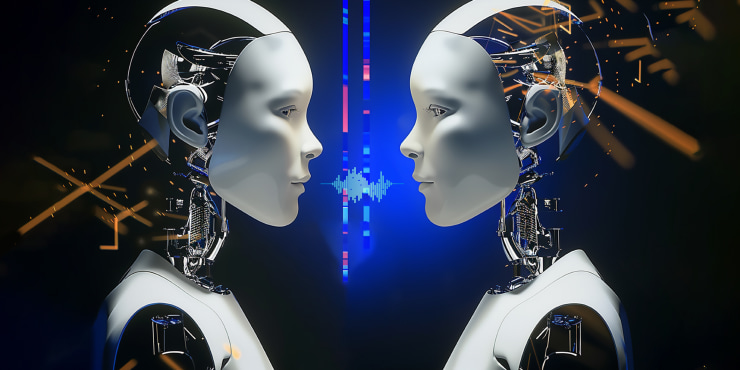
Thanks to the latest technologies, we can optimize and simplify all processes. Deep learning (DL) is a reformative force in AI, especially conversational ones. This combination of advanced algorithms and neural networks has created a real revolution. This redefined the field of human-computer interaction. Machines have overcome traditional limitations with DL, delivering natural and engaging dialogue. They were once believed to be the exclusive property of the people.
Let's explore how deep learning has become the cornerstone of modern conversational AI. It sets unprecedented standards for seamless communication between humans and machines. Delving into the core principles of deep learning and conversational AI is crucial. We lay the groundwork for an in-depth study of their symbiotic relationship and its profound implications. It is pivotal for the future of communication. Join us as we unravel the intricacies of this dynamic convergence. We uncover the insights it offers into the evolving nature of human interaction with technology.
Unpacking Deep Learning in Conversational AI
Deep learning lies at the heart of modern conversational AI. It orchestrates the intricate dance of dialogue between humans and machines. DL leverages neural networks, structures inspired by the human brain. It helps to mimic the nuances of natural conversation and comprehension. These networks process vast amounts of data through layers of interconnected nodes. They extract patterns and insights to facilitate more human-like interactions.
Era | Description |
---|---|
Rule-Based Systems (Pre-2000s) | Simple scripts governed interactions, limited in scope and flexibility. |
Statistical Methods (2000s) | Introduced probabilistic models to improve understanding but lacked contextual awareness. |
Neural Networks and Deep Learning | DL revolutionized conversational AI. It enables nuanced understanding and adaptive responses. |
It is also worth talking about crucial achievements in the field. It is because it is the achievements that show what results we can achieve:
- Natural Language Processing: DL models excel at parsing and comprehending human language. It allows for more fluid exchanges.
- Personalization: With deep learning, conversational AI systems can learn from user interactions. They tailor responses to individual preferences.
- Multi-Modal Integration: Integrating text, speech, and visual cues is crucial. It enables more effective and engaging interactions.
- Contextual Understanding: Deep learning enables AI to grasp context. It enhances dialogue coherence and relevance.
The evolution of conversational AI comes from rule-based systems. It affects models based on DL.
Conversational AI vs. Generative AI: Understanding the Distinction
Conversational AI vs. generative AI represent distinct facets of artificial intelligence. Each has its applications, capabilities, and contributions to AI-driven interactions.
Conversational AI:
- Application: Primarily focused on facilitating dialogue and interaction between humans and machines.
- Capabilities: Specializes in understanding natural language input. Also, it can generate appropriate responses to simulate conversation.
- Value: Enhances user experience by providing personalized, contextually relevant interactions. They are often used in chatbots, virtual assistants, and customer service applications.
Generative AI:
- Application: Centers around creating new content, such as images, text, or audio, often without specific input from users.
- Capabilities: Autonomously generate novel content based on learned patterns and styles. They foster creativity and innovation.
- Value: Empowers creativity to create rich, original content in art, music, and storytelling.
Deep learning vs. conversational AI:
- Enhanced Understanding: DL allows conversational AI systems to respond accurately to human actions.
- Human-Like Responses: DL algorithms analyze vast amounts of data. They enable conversational AI to generate responses. They mimic human speech patterns and expressions. It improves conversational quality and engagement.
Integrating deep learning further enhances the capabilities of conversational AI. It ushers in a new era of more natural and engaging human-machine communication. Understanding the distinctions between conversational AI vs. generative AI is crucial. It helps to leverage their unique strengths and applications in advancing AI-driven interactions.
Cost Analysis: Investing in Conversational AI
Investing in conversational AI entails various expenses, from initial setup to ongoing maintenance. Still, there are potential returns on investment and long-term cost-saving benefits. It makes it a compelling proposition. Initial setup of how much conversational AI costs:
- Development: Hiring skilled developers or teams to design and build conversational AI solutions. They can include natural language processing experts, machine learning engineers, and software developers.
- Data Acquisition: Acquiring and preprocessing large datasets for training the conversational AI model.
- Infrastructure: Provisioning servers, cloud services, or hardware. They are vital for deploying and hosting the conversational AI solution.
Ongoing maintenance costs:
- Updates and Upgrades: Regular updates to improve performance, add new features, and adapt to evolving user needs.
- Monitoring and Support: Continuous monitoring of system performance and user feedback analysis. It provides technical support.
- Data Management: Managing and updating training data is vital. It helps to ensure that conversational AI remains accurate and up-to-date.
Budgeting insights:
- Returns on Investment: Conversational AI can increase potency and improve client satisfaction. Also, it has moved to reduce operational costs, providing tangible returns on investment.
- Cost-Saving Benefits: Automating client service tasks, reduced response times, and scalability. It can lead to significant cost savings over time.
- Risk Mitigation: Budgeting for unexpected expenses and potential setbacks is crucial. It ensures smoother project implementation and mitigates financial risks.
Understanding how much conversational AI costs is vital for budgeting effectively. Also, it helps to realize the potential benefits of integrating AI solutions. The initial investment may seem significant. The long-term advantages are efficiency, client satisfaction, and cost reduction. This makes it a strategic investment for businesses aiming to stay competitive in the digital age.
Key Differentiators of Advanced Conversational AI Systems
It is pivotal to manage what a crucial differentiator of conversational AI is. They stand out from their counterparts through myriad distinctive features. Each contributes to a richer and more engaging user experience.
- Contextual Understanding: Advanced AI conversational systems excel at understanding and maintaining context. It is throughout a conversation. They enable more coherent and relevant responses.
- Emotion Detection: These systems can detect and interpret human emotions from text or speech inputs. They allow for more empathetic and tailored interactions.
- Personalized Responses: Tailoring responses to individual user preferences and past interactions. Advanced conversational AI systems create a more personalized and satisfying experience.
So, what is a crucial differentiator of conversational AI? Through the lens of deep learning, these differentiators are not just theoretical concepts. However, tangible advancements have reshaped the area of conversational AI. Deep learning has propelled conversational AI systems to unprecedented sophistication and effectiveness. They reform how humans interact with technology. It happens by enhancing contextual understanding, emotion detection, and personalization.
Implementing Deep Learning for Conversational AI
Integrating deep learning for conversational AI involves combining technical expertise and strategic planning. They help to achieve optimal performance and user satisfaction.
Technical considerations
In navigating the area of Conversational AI, delving into deep learning techniques is pivotal. Let's explore the technical intricacies of implementing Deep Learning for Conversational AI. It is generally based on understanding neural network architectures to optimize NLP models. We delve into critical considerations and best practices. They are vital for harnessing the power of deep learning in building conversational interfaces. Among them are:
- Data Acquisition: Gather diverse and representative datasets. They encompass various linguistic nuances and conversation scenarios.
- Model Architecture: Select appropriate deep learning architectures. RNNs, transformers, or sequence-to-sequence models tailored to conversational tasks are among them.
- Training Infrastructure: Ensure sufficient computational resources for efficiently training deep learning models. Consider factors like GPU availability and cloud computing services.
- Natural Language Processing: Employ pre-trained language models. Develop custom NLP pipelines for entity recognition, sentiment analysis, and intent classification tasks.
Strategic planning
This aspect is paramount, especially when integrating DL techniques. We delve into the intricacies of integrating deep learning for conversational AI systems. It is from understanding the fundamentals to navigating integration challenges. We explore critical strategies for leveraging deep learning. Among them are:
- Problem Formulation: Define clear goals and metrics to measure the success of your conversational AI system. They align with business goals and user expectations.
- Iterative Development: Adopt an iterative model training and refinement approach. They incorporate user feedback and performance evaluation at each stage.
- Ethical Considerations: Provide data confidentiality and prevent bias. Ensure the responsible use of AI throughout the life development cycle.
- Scalability and Maintenance: Design systems with scalability to accommodate future growth and updates. Establish robust maintenance protocols for ongoing performance optimization.
Challenges and best practices
Navigating the intricacies of DL integration for conversational AI presents challenges and opportunities. We delve into the complexities involved in harnessing DL techniques for Conversational AI. Also, we explore best practices to overcome obstacles and achieve optimal performance. Among them are:
- Data Quality: Ensure data quality and diversity to prevent bias and improve generalization.
- Overfitting: Regularize models and employ dropout and early stopping techniques to mitigate overfitting.
- Hyperparameter Tuning: Conduct systematic experimentation to find optimal hyperparameters for model performance.
- Evaluation Metrics: Utilize appropriate BLEU or F1 score to assess model effectiveness accurately.
Navigate technical complexities and strategic considerations, and install DL for conversational AI.
Future Directions: The Evolving Landscape of Conversational AI
The future holds immense potential for the evolution of conversational AI and DL. It implies upcoming technological innovations poised to redefine human-machine interactions. Also, conversational AI and deep learning unlock new applications across various domains. Among technological innovations are:
- Advanced Natural Language Processing: Future conversational AI systems will boast superior capabilities. They enable more seamless and intuitive interactions.
- Multi-modal Integration: Integrating text, speech, and visual input will create more engaging conversations. It leverages advancements in computer vision and speech recognition technologies.
- Personalization and Adaptability: AI systems will tailor responses to individual preferences. They leverage deep learning to learn from user interactions and adapt quickly.
- Businesses: Conversational AI will improve user engagement and revenue generation in specific sectors. These include user service, healthcare, and education.
- Consumers: Users will benefit from more personalized, intuitive interactions with AI assistants. They simplify tasks and enhance daily experiences.
- Ethical Considerations: Conversational AI and deep learning are becoming ubiquitous. They'll address data privacy, bias mitigation, and transparency issues.
- Fairness and Bias: It'll help to ensure equitable outcomes for diverse user groups.
- Privacy Preservation: Integrate robust measures. They'll help to protect user data and prevent unauthorized access or misuse.
Stakeholders must navigate the opportunities and challenges presented by these reformative technologies. Embracing ethical principles, fostering innovation, and prioritizing user-centric design is crucial. So we can harness its full potential of profound learning. It helps to drive positive societal impact and shape the future.