Redefining Virtual Assistance with Machine Learning
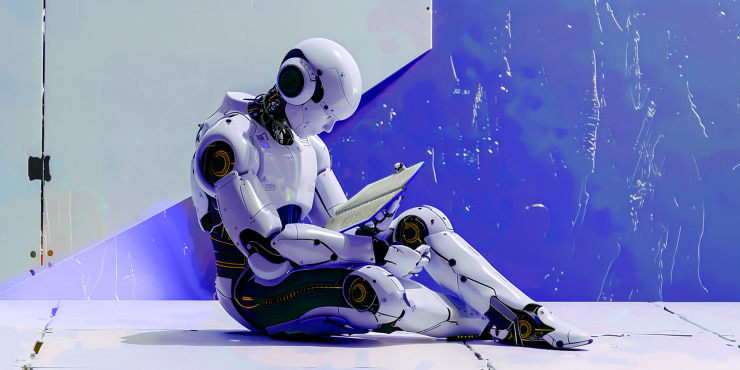
Digital workers have become ubiquitous. They're smoothly connected to our daily lives. They handle tasks and answer questions with ever-growing sophistication. Yet, their potential for even greater support lies on the horizon. ML offers a transformative key. It unlocks a new era of work with AI.
By combining the analytical muscle of machine learning with the accessibility and responsiveness of chatbots, we are witnessing a revolution in service automation.
This article delves into this exciting landscape. We will explore how ML is redefining digital help. We'll uncover how this powerful marriage transforms firms' connections with clients, changes experiences, and streamlines operations, ushering in a future of efficient service
The Evolution of Virtual Assistants Through Machine Learning
Bots started as text-based programs. Then, they have undergone a big metamorphosis. Clunky interfaces have given way to Siri, Alexa, and Google Assistant – intuitive companions that converse and adapt with us.
The magic of ML fuels this change. It empowers VAs to study our conversations, anticipate our demands, and respond surprisingly fluently. It's this integration that has redefined our relationship with techs.
VAs are no longer confined to simply following our commands and rules. They have become versatile web helpers. They constantly evolve alongside us. They shape the future of human-computer conversation.
Enhancing Responsiveness with Machine Learning
One of the contributions of ML to VAs is the improvement in responsiveness. ML analyzes vast amounts of our data. It includes past messages, searches, and our speech tone patterns. It allows voice assistants using machine learning to:
- Study from Dialogues. Training code enables VAs to refine their responses based on our feedback. Positive responses for specific phrasings or approaches encourage the VA to focus on those methods in the future.
- Adapt in Real-Time. Natural Language Processing techniques empower VAs to understand the context of our queries. It allows them to adapt their feedback dynamically. They account for sarcasm, humor, or frustration in the tone.
- Predict Demands. Predictive analytics based on our behavior enables VAs to anticipate demands. And they proactively offer help. It could involve suggesting tasks based on previous actions or reminding of events.
These advancements result in more natural and engaging communication for users. Voice assistants using machine learning can:
- Expect demands.
- Clarify ambiguous requests.
- Respond in a way that feels more like it's human.
Personalization at Scale
Another key area where ML shines is in personalizing experience. VAs can tailor their responses and functionality to individual demands. They analyze data such as preferences, location, and browsing history. It can include:
- Customized Recommendations. Voice assistant machine learning algorithms can personalize news updates. They offer music based on previous listening habits or recommend products.
- Context-Aware Help. Location-based services and calendar integration provide contextual assistance. Imagine receiving reminders for upcoming appointments or traffic updates. And it's all before you leave for work.
- Adaptive Interface. VAs can adapt their interfaces based on our preferences. It could involve changing the tone, adjusting the level of detail in responses, or prioritizing specific features on the interface.
The impact of personalization on satisfaction and engagement is undeniable. When a voice assistant with machine learning feels like it "understands you," it fosters trust. It encourages us to rely on it more heavily. It changes into increased engagement and, ultimately, a more positive brand experience.
However, there are ethical considerations on privacy and potential bias. These technologies evolve. So, it's vital to ensure responsible data collection practices and address potential bias in the training data.
Machine Learning in Voice Recognition Technologies
ML is the engine driving the remarkable progress in tone recognition. Let's delve into how ML tackles this issue and the breakthroughs it has enabled.
Applications of machine learning voice assistant:
- Feature Extraction. ML code analyzes raw audio data to extract key features. They are pitch, formants, and spectral information. These features help the model understand the underlying structure of speech.
- Acoustic Modeling. Statistical models predict the likelihood of specific sounds occurring in a sequence. It helps the system differentiate between similar-sounding words.
- Language Modeling. ML code analyzes large amounts of text. It's to understand the statistical relationships between words. It allows the tool to predict the most likely word sequence following a spoken phrase. It improves recognition accuracy in grammatically correct sentences.
Despite advancements in machine learning assistants, voice recognition still faces hurdles:
- Background Noise. Distracting sounds like music or traffic can interfere with accurate speech recognition.
- Accents and Dialects. ML tools trained on specific datasets may struggle with regional accents or pronunciation.
- Limited Context. Understanding the intent behind a spoken phrase can be challenging without extra context.
Breakthroughs in ML are addressing these challenges:
- Deep Training Techniques. Deep neural networks are showing remarkable progress in handling background noise. They also handle variations in speech patterns.
- Transfer Training. Pre-trained AI chatbot models with machine learning can be fine-tuned for specific accents or domains. It improves recognition accuracy for diverse customer groups.
- Contextual Awareness. Integrating VAs with other systems allows for the incorporation of contextual information. It's to enhance understanding of customer intent.
These advancements pave the way for even more natural and accurate tone communications. ML continues to evolve. So, AI bots will become adept at understanding complex requests.
Integrating Machine Learning with Conversational AI
Building sophisticated bots requires powerful synergy. It must be between conversational AI and ML. Let's explore how this integration fosters more natural and effective communications.
Conversational AI equips bots with the ability to understand and respond to human language. It includes:
- Natural Language Processing (NLP). NLP techniques enable VAs to analyze the intent behind queries. It's even when phrased ambiguously.
- Dialogue Management. Dialogue managers track conversation history and context. It allows VAs to maintain a coherent flow and avoid repetitive responses.
- Speech Recognition and Text-to-Speech. These technologies translate spoken input into text for processing. They generate natural-sounding responses back to the customer.
Machine learning provides the muscle that drives conversational AI functionalities. Here's how ML empowers bots:
- Training from Communications. Reinforcement training algorithms allow VAs to adapt their responses based on customer feedback. This training process improves the VA's understanding of customer intent and preferences.
- Personalization through Data Analysis. ML algorithms personalize the customer experience. They analyze data like search history, location, and previous communications. It enables VAs to expect demands, provide relevant suggestions, and adapt their communication.
- Advanced Natural Language Understanding. Deep training techniques enable VAs to grasp complex nuances of human language. It includes understanding sarcasm, humor, and cultural references. It results in more engaging and natural communications.
The seamless integration of conversational AI and machine learning empowers bots to:
- Handle Complex Requests. VAs can effectively navigate multi-step requests. They understand implicit information. Then, they offer solutions tailored to the customer's specific context.
- Provide a Natural Experience. By training and adapting to customer preferences, VAs can emulate human conversation patterns. It fosters a more natural and engaging communication.
- Continuously Improve Over Time. VAs collect more data and communicate with customers. So, the ML models underlying them continuously study and refine their capabilities.
This integration is paving the way for the future. There, bots are not just efficient tools. They are also companions, smoothly connected to our daily lives.
Future Trends and Possibilities in Machine Learning for Virtual Assistance
The coming age of bots shimmers with groundbreaking possibilities. The relentless march of ML ignites them. This transformative technology is on the cusp of fundamentally altering how we connect with computers.
We embark on a journey to uncover some of the most promising trends. They are trends that can unlock a revolutionary era of human-computer collaboration.
Fasten your seatbelts as we explore a future filled with bots. And it's worth making the important point that these bots don't just understand our needs. They anticipate them, changing the landscape of our work and lives forever:
- Enhanced Multimodality. Bots will move beyond tone recognition, integrating with other sensory inputs. Imagine using gestures, facial expressions, or eye gaze to communicate with your helper. Advancements in computer vision and natural interface design would facilitate this. It will allow for a richer and more intuitive experience.
- Deeper Contextual Understanding. Chatbot AI models with machine learning will become adept at grasping the broader communications context. It could involve incorporating information from calendars, emails, and other data sources. It's to anticipate demands and proactively offer help. Bots might suggest rescheduling a meeting based on a delayed email. Or they might offer relevant reminders based on upcoming travel plans.
- Emotion Recognition and Affective Computing. Machine learning could enable conversational AIs to understand more than just the content of queries. They also could understand the underlying emotional state. It would allow VAs to tailor their responses with empathy and adjust their communication style. Imagine a bot offering a calming tone during a stressful situation.
- Explainable AI and Trust. As ML models become more complex, ensuring transparency and customer trust will be crucial. Explainable techniques allow for understanding the reasoning behind a helper's response. It fosters a sense of control and builds trust in its capabilities.
- Lifelong Training and Collaboration. Future helpers will continuously study and evolve through customer communications. Imagine collaboratively training your VA on specific tasks or domains. It creates a personalized helper that caters to your unique demands and preferences.
These advancements in chatbot machine learning promise a future. There, bots become invaluable companions. They're smoothly connected to our lives, anticipating our demands and offering support.
Now imagine a world where your life is as simple as possible! You wake up, and a wakeful bot has already prepared your coffee by monitoring your sleep patterns. And as soon as you get out of bed, you can enjoy that flavorful beverage while the bot checks the weather. Now you can always wear clothes according to the weather, and never forget your umbrella! Remember, using robotization in our lives is not a replacement for human resources. It is a real opportunity to relax people's lives in this active time.