Exploring Generative AI: A Comprehensive Introduction
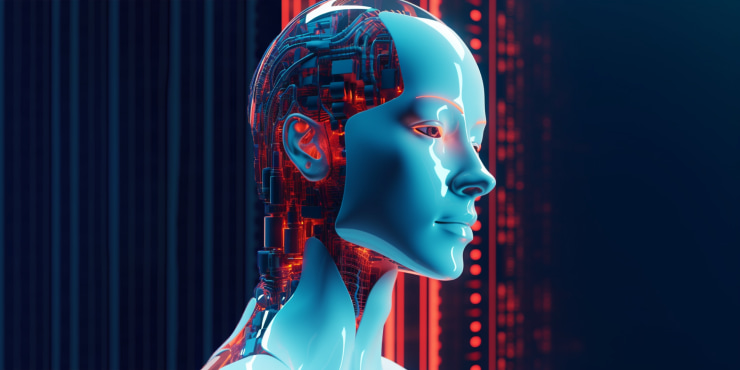
Within the realm of machine intelligence, a groundbreaking paradigm has captivated the creativity of researchers, developers, and enthusiasts — Creative AI. The importance of content-generating artificial intelligence continues to grow. It opens up new dimensions of creativity, problem-solving, and human-machine interaction.
We aim to demystify the concept of generative AI. We'll help you and shed light on its growing impact in the broader AI spectrum. We will also help you figure out generative AI vs predictive АІ.
Content-generating AI has a remarkable ability to create new material. This ability starts with images and text and ends with entire virtual environments. It is at the forefront of innovation, redefining what artificial intelligence can achieve.
Understanding the basic principles that distinguish generative AI from its counterparts is essential. Comprehension of its underlying mechanisms is very important. Our journey will cover various aspects of content-generating artificial intelligence. We will explore its role in art, language, and scientific research. Join us as we embark on this exciting exploration.
Generative AI vs. Predictive AI
In machine intelligence, Content-generating AI and Predictive AI represent two different paradigms. Predictive AI includes predictive analytics. It uses historical data to predict future trends, optimize processes, and assess risks. It is great for pattern recognition and data modeling. This makes it invaluable for decision-making in areas such as finance and business.
In contrast, Generative AI focuses on creativity. It uses models to generate new material, from images to text. This paradigm thrives on distributed learning. This allows you to produce original results outside of the training data. While Predictive AI makes decisions based on patterns, content-generating AI unlocks the potential to develop creative material.
Understanding Predictive AI
Predictive analytics is a subset of artificial intelligence. It involves the use of algorithms and statistical models to analyze historical data. This is important for predicting future events or trends.
Critical features of Predictive AI include:
- Pattern recognition. Predictive AI identifies patterns and trends in data sets. It relies on historical data to recognize correlations and relationships.
- Data modeling. Creates mathematical models based on historical data. This allows the system to understand the relationships between variables and make predictions.
- Prognostication. Predictive AI is used to predict future outcomes. This may include forecasting sales figures and share prices.
Judging by the name, this AI is used precisely for forecasting.
The Nature of Generative AI
Generative AI is designed to produce new material. It is not intended to predict existing patterns. Deep learning models, such as content-generating adversarial networks (GANs) and variational autoencoders (VAEs), form the foundation of it. The principles and capabilities of Generative AI include:
- Content creation. Content-generating AI can create new, original material.
- Imagination and creativity. It can imagine and produce new content. So, it demonstrates a certain level of creativity.
- Utilization in fine art and creative composition. Generative AI is often used in artistic fields. It creates unique works of art or design based on learned patterns.
In general, this is a new generation in the field of AI.
Comparative Analysis
This table summarizes the key differences between Generative AI vs Predictive AI.
Aspect | Predictive AI | Content-generating AI |
---|---|---|
Purpose | Predictions based on historical data. | Creation of new material, data, or art. |
Data Dependency | Heavily relies on historical data. | Learn underlying data distribution. |
Applications | Business analytics, finance, healthcare. | Creative fields, content generation. |
Creativity | Limited, based on existing patterns. | Exhibits creativity, generates novel material. |
Learning Approach | Pattern recognition, data modeling. | Learn data distribution for content generation. |
Examples | Recommender systems, fraud detection. | GANs for image generation, GPT for text generation. |
As a result, each type of AI has its own functions and purposes.
Generative AI in the Broader AI Context
Content-generating AI, in a broader context, is a paradigm shift. It focuses on creating new material and data. Unlike traditional AI models, which are often task-specific and follow rule-based approaches, Generative AI uses advanced deep learning techniques such as Generative Adversarial Networks and Variational Autoencoders to produce original results. Its application covers creative areas. These areas include art, music, and material creation. Thus, it demonstrates a departure from traditional approaches to problem-solving.
Generative AI vs. Traditional AI
Below we present a comparative table of generative АІ vs АІ.
Aspect | Content-generating AI | Traditional AI |
---|---|---|
Task Specificity | Broad focus on creativity, diverse outputs. | Designed for specific tasks, rule-based. |
Learning Approach | Learn from data distributions, imaginative. | Relies on explicit programming, predefined rules. |
Use Cases | Creative fields, material generation, art. | Automation, data analysis, decision-making. |
Adaptability | Adapts to a variety of problems. | Tailored to specific, predefined domains. |
Creativity | Introduces creativity and imagination. | Task-oriented, deterministic in nature. |
Examples | GANs for image generation, creative AI art. | Rule-based systems, traditional machine learning. |
So there is a significant difference between generative AI vs AI. From this comparison, we can see a considerable development of this technology.
The Evolution of AI
Generative AI marks a significant evolution in AI development. It represents a departure from the traditional task-oriented approach. This evolution is evident in creativity and imagination. In general, content-generating AI offers the following features compared to conventional AI:
- Introduces a level of creativity and imagination, going beyond the nature of traditional models.
- Learn from data distribution. It allows you to generate material not in the training data.
- Expands the scope of AI applications. It promotes creative fields, including art, design, and content creation.
- Demonstrates adaptability and ability to solve a broader range of problems.
Content-generating AI represents a new stage in the development of digital intelligence.
Generative AI and Conversational Interfaces
Generative AI is transforming conversational interfaces such as chatbots and virtual assistants. It imbues them with a fine-grained сomprehension of natural language. They can generate relevant responses. In Conversational AI, the synergy with content-generating AI introduces a dynamic layer into the interaction. Generative AI capabilities allow chatbots to generate human responses. They adapt to various user requests and the evolving context. This not only improves user engagement. It also facilitates more personalized and efficient problem-solving. Content-generating AI integration improves conversational interfaces. It makes them adept at imitating the subtleties of human conversation. Developers produce Conversational AI in this manner.
The Role of Conversational AI
In the question of generative АІ vs conversational АІ, the second depends on the first. This is a new stage of AI development.
Conversational AI refers to applying artificial intelligence technologies to create human interaction. This interaction occurs between machines and users using natural language. The primary purpose of conversational digital intelligence is to imitate human speech. For this, developers use advanced chatbots or even digital employees. This AI uses natural language processing (NLP) and machine learning to understand user requests. Conversational digital intelligence finds application in customer support and automation. It offers seamless and convenient interaction.
Integrating Generative AI with Conversational Systems
Content-generating AI is critical in improving conversational applications. It provides chatbots and virtual assistants the following benefits:
- Natural language generation (NLG). It allows you to produce human-like responses and material.
- Contextual understanding. It improves the ability of conversational systems to understand and respond contextually.
- Creating dynamic content. Generative AI allows chatbots to generate responses dynamically.
- User engagement. It promotes a richer and more interactive discussion, engaging users.
- Problem solving. It helps solve problems by generating informative, context-sensitive answers.
Integrating generative AI vs conversational AI systems improves the overall user experience. This makes the interaction more dynamic, personalized, and effective.
Generative AI for Marketing
Content-generating AI is revolutionizing marketing strategies. It introduces innovative and dynamic material creation capabilities. In marketing, this technology allows you to produce attractive and personalized content. For example, Generative AI can create tailored ad copy, design graphics, and even produce marketing campaigns. This not only optimizes the creative process but also improves interaction. Chatbots based on content-generating AI further contribute to marketing efforts. They provide interactive and personalized interaction with customers. They respond to inquiries and guide users through information about a product or service. Overall, Generative AI for marketing promotes efficiency, creativity, and a personalized approach. This allows companies to communicate with their audiences in new and effective ways.
AI-Generated Content: The New Frontier
AI-generated material represents a breakthrough, transforming the landscape of creativity and communication. It uses advanced algorithms, including GANs and language models, such as GPT. Artificial intelligence autonomously creates contextually relevant text, images, and video content. This paradigm shift provides unparalleled efficiency and scalability. This, in turn, affects digital media, marketing, and more. AI can quickly generate large volumes of material and adapt to different styles. This testifies to the transformative potential of digital intelligence. As this new frontier unfolds, it brings both opportunities and challenges.
What is AI-Generated Content?
AI-generated material refers to creative material. It can be text, images, videos, etc., produced by digital intelligence algorithms. Artificial intelligence uses deep learning models to mark a paradigm shift in content creation. They help the autonomous creation of diverse and contextually relevant material. Its value lies in efficiency, scalability, and creativity. It brings them into the content creation process. AI can quickly create large volumes of material and adapt to different styles. It can even customize output based on specific criteria. This, in turn, is changing the landscape of digital media and marketing.
The Impact of AI-Generated Content
The impact of AI-generated material is profound. It opens both opportunities and problems.
AI accelerates content creation by reducing time and resource constraints. However, concerns exist about the potential oversaturation of general material and the loss of the human touch. Artificial intelligence also facilitates creativity and allows customization for specific audiences. However, it is essential to balance creativity with ethical considerations. It is also vital to ensure that AI-generated content complies with ethical standards.
Digital intelligence adapts material to individual preferences, improving interaction with the user. But next to this, ensuring the responsible use of personal data is essential.
So, what is AI-Generated Content? In summary, AI-generated material is breaking new ground with colossal potential. But at the same time, it requires careful consideration of ethical, creative, and social consequences.
Conclusion
This research delves into content-generating AI, conversational interfaces, AI in marketing, AI-generated material, and the broader AI landscape. Generative artificial intelligence has become a creative force. It has revolutionized material creation and communication. Conversational AI, driven by content-generating AI, improves user interaction. And digital intelligence in marketing optimizes creativity and personalization. The emergence of material produced by machine intelligence marks a transition point. This opens up both possibilities and ethical considerations. As these technologies evolves, it is imperative to be responsible for their impact. Harmonious cooperation between human ingenuity and artificial intelligence should be promoted. The dynamic interaction of these achievements leads us into the future. АІ continues to change how we create, communicate, and interact with the digital world. Implement all its advantages in your business today!