MIT Breakthrough: System Allows Robots to Identify Object Properties Through Handling
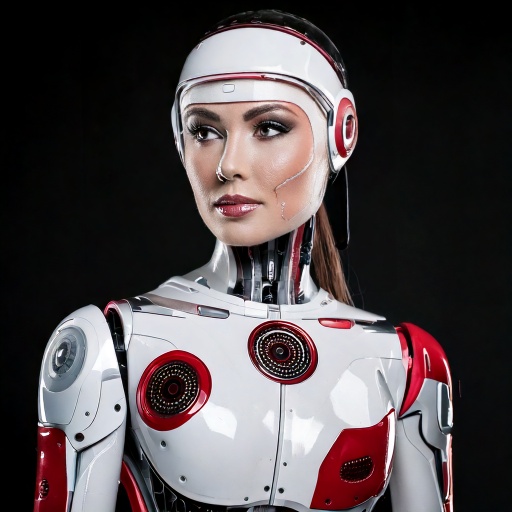
On May 8, 2025, researchers from MIT, Amazon Robotics, and the University of British Columbia introduced a pioneering system that allows robots to determine an object's physical properties—such as weight, softness, and internal contents—by simply picking it up and gently shaking it. This advancement marks a significant step toward more autonomous and adaptable AI employees in various industries.
Key Features and Significance:
- Internal Sensor Utilization: The system employs internal sensors, eliminating the need for external measurement tools or cameras.
- Simulation-Based Learning: A novel simulation method enables robots to rapidly identify object characteristics through proprioception, mimicking human tactile sensing.
- Practical Applications: Particularly beneficial in environments where visual data is limited, such as dark basements or disaster-stricken areas.
- Cost-Effective: The approach offers a low-cost alternative to traditional methods that rely on computer vision.
Implications for Non-Human Workers
This development enhances the capabilities of non-human workers, enabling them to perform tasks that require an understanding of object properties without relying on visual inputs. Such advancements are crucial for tasks in challenging environments, where traditional methods may fall short.
The introduction of this system signifies a major leap forward in robotics, allowing AI employees to interact with their environment in a more intuitive and efficient manner. As robots become increasingly adept at understanding and manipulating objects, their role in various industries is set to expand, offering solutions to complex challenges.
Key Highlights:
- Innovative System: MIT's new system enables robots to identify an object's properties, such as weight, softness, and internal contents, by simply handling it.
- Internal Sensors: The system uses internal sensors, reducing the need for external tools or cameras.
- Simulation-Based Learning: Robots learn to identify object characteristics through proprioception in a simulated environment, mimicking human tactile sensing.
- Cost-Effective Solution: The system offers a low-cost alternative to traditional computer vision methods for object identification.
- Practical Use Cases: Particularly beneficial in environments where visual data is limited, such as dark or disaster-affected areas.
- Impact on Non-Human Workers: The development enhances the capabilities of AI employees, making robots more adaptable and efficient in handling tasks in complex environments.
Reference:
https://news.mit.edu/2025/system-lets-robots-identify-objects-properties-through-handling-0508