Overcoming AML Transaction Monitoring Challenges with AI
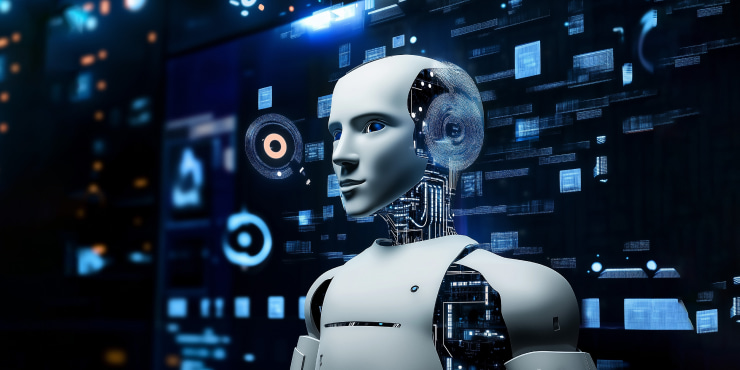
Did you know money laundering accounts for 2% to 5% of global GDP? It’s equivalent to approximately 2 trillion dollars every year. In the fight against these financial crimes, identity verification processes are fundamental. Anti-money laundering (AML) is a regulatory requirement and a powerful tool. It helps to prevent terrorist financing and other illegal activities. We will analyze in detail the importance of AML in today's financial landscape. Are you ready to take your knowledge of identity verification to the next level?
Automated transaction monitoring is a perfect solution that leverages the latest technologies. Artificial intelligence (AI) transaction monitoring can analyze vast transaction data. This occurs at incredible speed and accuracy. It detects complex patterns. Traditional methods usually miss them. This modern tactic enhances the effectiveness of AML efforts and reduces false positives.
Challenges in AML Transaction Monitoring
What is AML transaction monitoring? It faces several significant challenges. They hinder its effectiveness in identifying suspicious activities:
- High volumes of false positives: Standard systems often flag legitimate transactions as suspicious. They result in overwhelming numbers of alerts that need manual review. This causes inefficiency and increases operational costs.
- Regulatory compliance: Financial institutions must adhere to strict regulatory requirements. It varies across regions. Staying compliant with evolving laws adds complexity to AML efforts. Systems need constant updates to remain effective.
- Evolving money laundering techniques: Criminals continually adapt their methods. They bypass traditional monitoring systems. Criminals use more sophisticated strategies, including layering, structuring, and using complex financial instruments. These techniques make detection more difficult.
Rule-based systems rely on predefined conditions and thresholds to flag suspicious activities. They often fall short in detecting these complex laundering schemes. Such systems lack the flexibility to adapt to evolving patterns of illicit behavior. For instance, AML transaction monitoring scenarios may become more challenging to recognize. Fraudsters use legitimate transactions to disguise illegal ones.
Traditional AML systems are resource-intensive. They require significant human intervention for monitoring, investigation, and reporting. This labor-intensive process is costly and slow to adapt to new threats. The inefficiency of rule-based systems is essential. It is combined with the rapid pace of financial crime evolution. It underscores the need for more adaptive and advanced solutions, including AI transaction monitoring alerts. It can automate, refine, and accelerate the detection process.
High False Positives and Operational Inefficiency
Traditional transaction monitoring systems often generate a high volume of false positives. They flag legitimate transactions as suspicious. These excessive alerts result in unnecessary investigations. They lead to significant inefficiencies within the compliance process. The primary reasons for this issue include rigid rule-based frameworks. They trigger alerts based on pre-set criteria. It happens without accounting for the nuances of individual transactions.
The impact of false positives on operational costs is profound. Compliance teams are tasked with manually reviewing these alerts. They consume valuable resources and time. AML challenges can lead to:
- Increased labor costs: Large volumes of alerts require significant human intervention. They drive up costs for financial institutions.
- Delayed response times: The backlog of false positives is impactful. It can set back the investigation and resolution of legitimate suspicious activities.
- Compliance risks: Critical suspicious transactions may be missed amidst false positives. Institutions could face regulatory penalties or reputational damage.
These challenges highlight the limitations of traditional monitoring systems. They underscore the need for more advanced technologies to streamline the detection process. AI-driven systems reduce false positives and offer more precise and efficient bank transaction monitoring. Such systems allow compliance teams to focus on actual risks.
Difficulty in Detecting Complex Patterns
Rule-based systems in transaction monitoring face significant challenges when dealing with detecting advanced money laundering schemes. This is because they rely on fixed criteria and patterns. These systems are unable to adapt to the increasingly sophisticated techniques employed by criminals to hide illicit activity. For example:
- Layering: This technique involves the process of moving funds. It happens through many transactions or accounts to obscure their origin. Traditional systems follow simple rules. They often fail to spot these complex transfer patterns.
- Integration: Integration occurs when laundered money is reintroduced into the financial system through seemingly legitimate transactions such as large purchases or investments. Rule-based systems struggle to differentiate between legitimate and suspicious activities.
- Cross-border transactions: They are another method used to evade detection. Criminals can move funds across multiple jurisdictions. They exploit differences in regulations and delays in information sharing. Traditional monitoring systems struggle to track these international transactions in real time.
These AML challenges highlight the need for more advanced solutions. Rule-based systems cannot keep up with these dynamic methods of money laundering. They create gaps in detection that modern AI solutions can address. Such systems enable more effective transaction monitoring.
How AI Transforms AML Transaction Monitoring
AI reforms transaction monitoring. It leverages ML and predictive analytics to enhance the detection of financial crimes. Traditional rule-based systems are limited regarding their ability to adapt to evolving patterns of illicit activity. Whereas AI can continuously learn and improve from vast amounts of data. It enables a more accurate and dynamic approach. It helps with detecting suspicious transactions.
One of AI's primary strengths is in crime bank transaction monitoring. This is its ability to process and analyze massive datasets in real-time. Such a capability allows AI systems to:
- Detect hidden patterns: AI identifies complex relationships within transaction data. It may go unnoticed by traditional systems. AI recognizes subtle anomalies. It can flag potentially fraudulent activities more effectively.
- Reduce false positives: AI distinguishes between legitimate and suspicious transactions more accurately. It significantly lowers the number of false positives and minimizes unnecessary manual reviews.
- Provide real-time monitoring: AI can analyze transactions as they happen. It enables financial institutions to respond immediately to suspicious activity. This is rather than after the fact. It is crucial for preventing the movement of illicit funds. It is possible to do this before they can be fully integrated into the system.
- Dynamic risk scoring: AI continually adjusts them. Risk scores are based on up-to-date transaction data and historical patterns. This helps create more accurate risk profiles for clients. AI improves decision-making and compliance.
Using ML and predictive analytics is crucial. AI empowers financial crime transaction monitoring to evolve in real time. It provides a more efficient and adaptive defense against increasingly sophisticated criminal tactics. The integration of AI is revolutionizing how financial institutions approach AML. This makes it faster, more precise, and better suited to handle the complexities of modern financial crime.
AI Techniques for Improved AML Monitoring
AI has revolutionized the AML transaction monitoring process. It integrates several advanced techniques that significantly enhance the detection of suspicious activities. These technologies allow financial institutions to identify hidden risks more accurately and efficiently. This is in comparison to traditional rule-based systems:
- ML for anomaly detection: These algorithms excel at analyzing large datasets. They identify unusual patterns. Rule-based systems rely on predefined thresholds. ML can detect deviations from normal behavior. This is possible even when those anomalies are subtle or complex. For instance, it can spot outliers in transaction volume or frequency. It may indicate money laundering. They include layering or structuring of transactions.
- Natural language processing (NLP): This technology is essential for transaction monitoring AML. It enables AI systems to process unstructured data. They include text-based customer communications, emails, and news reports. AI analyzes this unstructured content. It can uncover additional indicators of financial crime. It may not be immediately evident in transaction data alone. NLP can help identify fraudulent schemes through keywords or patterns in customer interactions. They align with known money laundering tactics.
- Behavioral analytics: This technology identifies deviations in customer activity. AI uses it to establish a baseline of regular customer activity. Then, it flags any significant deviations from that pattern. This technique helps detect suspicious behavior. It may be unusual withdrawal amounts, transfers to high-risk countries, or sudden spikes in activity. They don't match the customer's historical behavior.
These techniques improve the accuracy of the transaction monitoring process in AML. They enable faster identification of potential threats and reduce false positives. Such technologies automate time-consuming tasks. Ultimately, these innovations enhance the overall effectiveness of the AML transaction monitoring process. They ensure a more robust defense against financial crimes.
Overcoming Implementation Challenges of AI in AML
Implementing AI in AML processes presents several challenges. Financial institutions must address them to unlock the full potential of this technology. Key barriers include data quality, integration with legacy systems, and regulatory concerns:
- Data Quality: AI models rely heavily on comprehensive, clean, accurate data. Poor data quality can lead to inaccurate predictions and false positives. They also cause missed suspicious activities. To mitigate this, organizations should invest in robust data pipelines. It ensures data collection, cleansing, and normalization across all channels.
- Integration with Legacy Systems: Financial institutions often rely on them. Outdated systems may not be compatible with advanced AI models used for bank transaction monitoring. The solution lies in incremental integration. This means upgrading or modernizing legacy systems in stages. Ensuring seamless communication with new AI platforms is crucial. This approach minimizes disruption to daily operations. It provides a smooth transition to more sophisticated, AI-driven monitoring systems.
- Regulatory Concerns: Compliance with ever-evolving regulatory requirements is critical in the financial sector. AI must be used to meet local and global standards, including GDPR or the USA PATRIOT Act. This can be addressed by adopting explainable AI models. They provide transparency into how decisions are made. These models ensure accountability and alignment with regulatory frameworks. They address potential issues that arise with manual transaction monitoring. It may lack the same level of transparency and efficiency. Financial institutions must integrate AI. They can improve the effectiveness and regulatory compliance.
- Aligning Initiatives with Regulatory Frameworks: Financial institutions must align their tools with them. It helps to ensure compliance. This alignment fosters trust with regulators and avoids potential fines or legal challenges.
The difference between transaction screening vs. transaction monitoring is key here. The first one identifies suspicious transactions based on known patterns. Transaction monitoring is an ongoing process of tracking transactions in real time. It helps to detect emerging risks. Both must be integrated to ensure adequate AML controls. Relying on transaction monitoring alone is no longer sufficient. AI-enhanced systems offer much-needed scalability.
The Future of AI in AML Transaction Monitoring
The future of AI in AML transaction monitoring holds immense promise. Evolving technologies continue to shape how financial institutions combat money laundering and how financial crimes are committed. Several trends are likely to emerge in the coming years transforming how banks and regulatory bodies detect and prevent illicit activity:
- AI Risk Prediction Models: One of the key innovations in AML transaction monitoring is their development. These models will identify suspicious patterns. They also predict potential risks based on historical data and emerging trends. This proactive approach will improve the ability to prevent financial crimes before they occur. They offer banks a more dynamic and forward-looking monitoring system.
- Blockchain-Based AML Systems: Another trend is the integration into transaction monitoring in bank systems. Blockchain’s transparency and immutability can offer secure, real-time tracking of transactions. They make it easier to trace suspicious activities across borders. The decentralized nature of blockchain also improves data integrity. It reduces the risk of tampering.
- Staying Ahead of Financial Crime Through AI Innovation: Staying ahead of sophisticated criminal techniques requires banks to invest in AML transaction monitoring solutions. They are agile, adaptable, and capable of learning from emerging threats. Financial institutions will strengthen their compliance efforts by embracing AI and related technologies. They will also play a critical role in protecting the global financial ecosystem.
- Fully Automated AML Platforms with Continuous Learning: AI and ML evolve. AML transaction monitoring platforms will become fully automated with continuous learning capabilities. These platforms will adapt and improve based on new data enabling them to detect novel money laundering tactics. Over time, this system will minimize the need for human intervention. It will significantly improve transaction monitoring.
The future of AI will be revolutionary. AML has become an indispensable tool in the fight against financial crime. In addition to serving as a regulatory requirement, it is a fundamental process. It lays the foundation for effective prevention of money laundering and terrorist financing. Follow us to be a digital guru.