Explanation of How AI Сonsumes Water
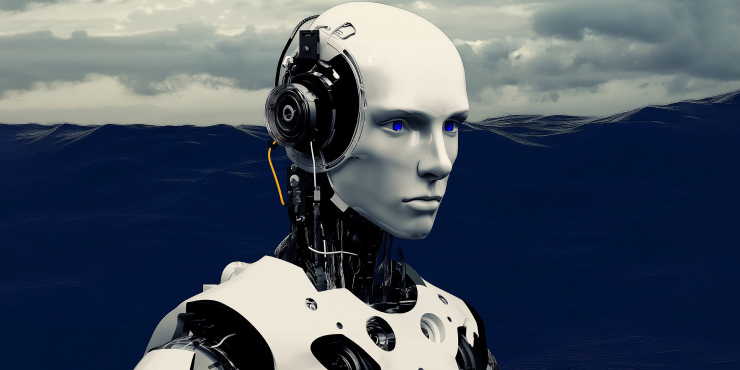
Artificial intelligence (AI) continues to revolutionize industries. Its impact on the environment is becoming a growing concern. One of the most pressing issues is AI water usage. Its computational demands of it require significant amounts of energy and resources, especially concerning data centers and the training of large models. Among these, water is crucial in cooling the infrastructure that powers AI operations. Data centers, which house the servers used for AI tasks, are notorious for their high water consumption.
Cooling systems often rely on water to prevent overheating. AI becomes more advanced and widespread. The demand for computational power is escalating. It leads to an increase in water usage. This has raised concerns about the sustainability of AI in environmental resources. We will explore how AI consumes water and the environmental impacts. Our team also prepares potential solutions for you. These solutions aim to reduce water usage in AI operations.
Understanding AI’s Resource Footprint
AI has an undeniable environmental footprint. This is primarily due to its high energy consumption and the resources. They are required for its operations. One of the most overlooked aspects of this is how AI takes water from natural sources. It is especially important in data centers. They are typically powered by AI computations. Such centers rely heavily on water to cool their systems. AI, with its complex algorithms and large-scale models, demands extensive computational power. It generates a substantial amount of heat.
Data centers utilize cooling systems that often depend on water. They prevent overheating and ensure servers run at optimal conditions. How AI water from cooling towers and chillers is critical. It maintains the temperature of these systems. The water used is typically recycled. Its overall demand increases as the scale of AI infrastructure grows.
The relationship between computational demands and heat generation creates a cycle. It requires more energy and water to sustain AI systems. AI models become larger and more sophisticated. The strain on data centers increases. It leads to even higher water and energy consumption.
The broader environmental impact includes:
- Energy consumption: AI requires significant energy for computing power and cooling systems.
- Water use: Data centers rely on water for cooling. There is an increasing demand on local resources.
- Heat generation: The higher the computational activity, the greater the heat output. It necessitates more cooling efforts.
Finding ways to mitigate AI’s environmental footprint is crucial for sustainable development. This includes reducing water usage in data centers.
The Role of Data Centers in AI Water Consumption
Data centers are critical in water AI consumption. They rely on it for cooling systems. It helps them to prevent overheating of the servers that power AI operations. These cooling systems typically use large amounts of water. This is typically circulated through cooling towers or heat exchangers. They maintain the optimal temperature of the equipment.
For instance, a typical data center can consume millions of gallons of water annually. It supports its cooling systems. How much water does ChatGPT use is a related question. Large AI models require extensive computational resources. They contribute to higher water consumption in data centers. There, they are typically hosted. Although specific data on AI models like ChatGPT is not always disclosed. Data centers can use up to 1.5 million gallons of water per day in some regions.
Key factors include:
- Cooling requirements: High-performance computing generates significant heat. It necessitates water cooling.
- Scalability: The larger the data center, the greater the water consumption.
- Geographical location: Water availability can vary by region. It impacts the overall consumption.
AI Model Training and Water Usage
Training large AI models significantly increases computational demands. It, in turn, raises the need for water consumption. The training process involves running vast amounts of data through complex algorithms. They require powerful servers to process and store this information. These systems work at full capacity. They generate considerable heat, necessitating cooling systems to maintain operational temperatures.
Model training involves several phases that demand high computational resources:
- Data Preprocessing: Preparing and cleaning large datasets for training models. They can increase computational load and energy usage. It impacts finding water AI solutions.
- Pre-training: Models process massive datasets. They require intense computational power. It drives higher energy and water consumption for cooling.
- Fine-tuning: Refining models with specific datasets consumes substantial resources. This is crucial even after initial training.
- Model updates: Regular retraining of models also requires significant energy and cooling. It further increases water usage.
How much water ChatGPT uses per day is typically linked to the scale of the data centers hosting the model. For instance, some large data centers may use up to millions of gallons of water daily to cool servers. Key factors include:
Factor | Impact on Water Usage |
Training Phases | High computational demands lead to increased cooling requirements. |
Scale one Data Centers | Larger models in massive data centers result in greater water consumption. |
Server Density | Higher server density in AI operations leads to increased cooling needs. |
How AI is taking water during training processes is a critical environmental consideration. AI technologies continue to scale.
Factors Influencing AI’s Water Consumption
AI’s water consumption is influenced by several factors. These factors affect the scale and efficiency of water usage in data centers. These factors include geographical location, cooling technology, and the type of energy used. It powers the servers. Here are the factors that influence AI for water:
- Geographical Location: The location of data centers is significant in their water usage. Some areas have access to abundant freshwater resources. They may rely on water-based cooling systems more heavily. Conversely, regions experiencing water scarcity may implement more sustainable solutions. They will mitigate environmental impact. For example, data centers in hot, dry climates often require more intensive cooling. They increase water consumption.
- Type of Cooling Technology: Traditional methods of AI finding water are evaporative cooling. They rely heavily on water to absorb and dissipate heat from servers. This method is effective but can be resource-intensive. In contrast, newer technologies reduce the need for water. These are liquid or direct-to-chip cooling. Such techniques use liquids other than water or targeting heat directly at the chip level. It minimizes the cooling needs of the overall system.
- Energy Sources: The type of energy powering a data center can also impact water usage. Traditional fossil fuel-powered plants often consume large amounts of water for cooling. In contrast, renewable energy sources typically have lower footprints. These are solar or wind. They reduce the overall environmental impact of AI operations.
How much water does ChatGPT use per day? The overall amount can be influenced by these factors. AI operations can minimize their water footprint efficiently. They may still maintain high-performance levels.
Climate and Regional Variations
The climate and location of data centers significantly influence their water consumption. Environmental factors dictate cooling requirements and available resources. There are key regional and climate-based impacts on data center water use:
- Hot and Arid Regions: Data centers in deserts climates consume more water for cooling. This happens due to higher ambient temperatures. Evaporative cooling systems rely on large volumes of water. For instance, facilities in Nevada or the Middle East may face challenges. They balance operational needs with local water scarcity.
- Cold and Temperate Regions: Data centers in cooler climates benefit from free cooling. These are Scandinavia or Canada. They use naturally cold air or AI for water to regulate temperatures. These locations can significantly reduce their reliance on water-intensive cooling methods.
- Coastal Areas: Facilities near oceans can use seawater for cooling, minimizing freshwater usage. However, processing seawater involves challenges. They are corrosion and additional energy for desalination.
- Urban vs. Rural Settings: City centers often have higher cooling demands. This happens due to heat island effects, increasing water usage. Rural areas, with access to natural cooling sources like rivers, may use less water.
Data centers can optimize water use by leveraging AI technologies. This is based on climate and regional conditions.
Cooling Technologies and Innovations
Advancements in cooling technologies are transforming how data centers manage heat. They address water consumption concerns. There are some innovative cooling solutions. What machines use the energy of evaporating water to generate power? Let’s discuss:
- Liquid Cooling Systems: They use liquid coolants. These are water or specialized fluids. Such systems transfer heat more efficiently than traditional air cooling. Direct-to-chip cooling circulates liquid directly over processors. It reduces the need for large-scale water-based systems.
- Closed-Loop Cooling: Closed-loop systems recycle water within a sealed environment. They significantly lower water wastage. These systems reduce dependency on external water sources. They make them ideal for regions with limited water availability.
- AI-Driven Cooling Optimization: AI monitor and manage cooling systems. It ensures optimal performance while minimizing water and energy use. Finding water AI tools predict cooling needs. They are based on operational and environmental factors.
- Free Cooling Technologies: They leverage naturally cool air or water from local environments. It maintains server temperatures. Examples include data centers in cold climates using ambient air facilities utilizing seawater for cooling.
Environmental Impacts of AI Water Use
Excessive AI water use has significant environmental impacts. This is particularly on ecosystems and local water supplies. Here are some key consequences:
- Strain on Local Water Supplies: AI data centers often draw large amounts for cooling, depleting resources. This is important in regions with limited water availability. This can lead to competition for water between industries and local communities. It creates social and economic tensions.
- Ecosystem Disruption: Excessive water extraction may lower water levels in rivers and lakes. It affects aquatic habitats and species. Warm water discharged from cooling systems can alter local ecosystems. It leads to thermal pollution that harms aquatic life.
- Indirect Use of Water in Energy Production: Many data centers rely on electricity from power plants. They consume water for cooling. This creates a ripple effect. It compounds environmental pressures across multiple sectors.
- Climate Impact Amplification: Over-reliance on water-intensive cooling systems exacerbates droughts. This happens in regions with warm climates.
Mitigating these impacts requires adopting sustainable cooling technologies and requires prioritizing resource-efficient practices.
Solutions and Strategies for Sustainable AI
There are solutions and strategies to mitigate AI’s water consumption. They also promote sustainability. Let’s explore them:
- Efficient Cooling Technologies: Transitioning to advanced systems reduces water dependency. These are liquid cooling and closed-loop cooling. Such technologies recycle water and minimize wastage. They offer a sustainable alternative to traditional cooling methods.
- Renewable Energy Integration: Utilizing solar, wind, or hydroelectric energy is essential to lower reliance on water-intensive energy production. Renewable energy sources also reduce carbon emissions. They create a dual environmental benefit.
- Optimized Data Center Designs: Their strategic placement in cooler climates is essential. It reduces the need for extensive cooling systems. Improved airflow designs and energy-efficient equipment further minimize resource use.
- Exploring Innovative Technologies: Cool water powers rely on its natural cooling properties. They offer eco-friendly solutions. Such technologies present innovative ways. They reduce energy demands while cooling AI systems.
Adopting these measures ensures sustainable growth in AI. They safeguard vital environmental resources.
Future of AI and Environmental Responsibility
Balancing the rapid growth of AI’s water and energy demands with eco-sustainability is crucial. It provides a greener future. How can this balance be achieved? Let’s discuss:
- Ongoing Research: Scientists are developing energy-efficient AI algorithms. They minimize resource use during training and operation. Alternative cooling systems and optimized hardware design are helpful. They will reduce dependency on water-intensive processes.
- Policy Considerations: Governments and environmental organizations are drafting regulations. They will limit the environmental impact of data centers. Incentives for adopting sustainable practices encourage industry leaders to embrace greener technologies.
- Industry Commitments: Companies are committing to transparency. They report how AI water is typically utilized in their operations. Major tech firms are investing in renewable energy and carbon-neutral facilities. They are also funding water recycling systems.
- AI for Sustainability: AI technologies address environmental challenges ranging from optimizing water distribution systems to monitoring ecosystems. Innovative solutions help industries adopt sustainable practices.
The AI industry prioritizes efficiency, innovation, and collaboration. It can evolve responsibly. This ensures its growth does not come at the expense of the planet. Looking ahead, a sustainable future for AI is achievable with collective action. It will significantly focus on environmental stewardship. Contact Newo.ai to get professional guidance in the digital world.