Deciphering AI, Machine Learning, Deep Learning, and Neural Networks: A Comparative Analysis
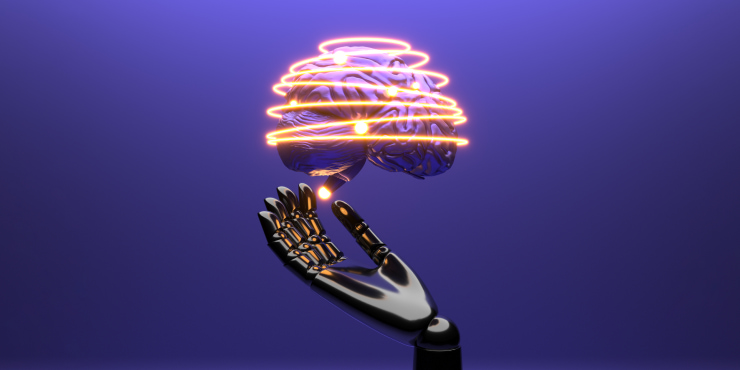
Within the complex realm of contemporary tech, expressions are commonly employed. Among them are AI, ML, DL, and NN. But, they can be easily entangled in confusion. Each term represents a crucial facet of technological advancement. Yet, they possess unique characteristics that set them apart. This article begins to unravel these concepts' interconnected yet distinct nature. The aim is precise: to demystify and compare them. By clarifying each role, we aim to empower readers with a more profound comprehension.
We foster a nuanced appreciation of these reformative elements shaping our World of the Web. Join us in exploring the intricacies of these techs. It'll help you better navigate the developing MI sector and its derivatives. Our comparative analysis will be helpful for you to make the first step to your prosperity. Let’s grub into the subsets of artificial intelligence. Discover all these hi-tech concepts and their particular qualities with us.
Understanding the Subsets of Artificial Intelligence
We delve into knowledge engineering. Rummaging into its subsets is crucial for a comprehensive grasp of the field. Subsets like ML, Deep Learning, NLP, and others each play unique roles, contributing to the broader area of intelligent systems. This exploration facilitates a nuanced understanding of how distinct methodologies within AI coalesce. It enables advancements that redefine the capabilities and apps of intelligent technologies.
The Spectrum of AI
AI is a comprehensive umbrella term. It encapsulates a broad spectrum of technologies designed to mimic human intelligence. Within this expansive domain, various subsets play distinctive roles. Let's unravel the spectrum:
- Artificial Narrow Intelligence. Specialized in executing specific tasks, lacking general cognitive abilities.
- Artificial General Intelligence. Possesses human-like cognitive capabilities, exhibiting intelligence across diverse domains.
- Machine Learning. Utilizes algorithms, enabling systems to learn and adapt from data.
- Deep Learning. A subclass involving computer-aided learning with multiple layers for intricate pattern recognition.
- Natural Language Understanding. It enables machines to comprehend and generate human-like text.
Comprehending the spectrum of MI and its subset provides insight into various techs.
Machine Learning and Deep Learning as Subsets
These subclasses carve distinct niches. They are contributing significantly to the evolution of intelligent systems. Here's a breakdown of such subsets of artificial intelligence roles within the broader AI framework:
- Machine Learning:
- Utilizes algorithms, enabling systems to learn and adapt from data.
- Involves diverse approaches, including controlled and uncontrolled learning.
- Deep Learning:
- A specialized subset within ML, employing a neural net with multiple layers.
- Excels in complex pattern recognition and decision-making tasks.
- Neural Net Architecture:
- In DL, they comprise interconnected layers, mirroring hierarchical learning.
- Pattern Recognition:
- ML focuses on various pattern recognition tasks. Deep learning excels in intricate, hierarchical pattern recognition.
- Adaptive Learning:
- ML and deep learning exhibit adaptive learning, refining systems based on experience.
Understanding how these subgroups fit within the broader AI framework is pivotal. It unveils their specialized roles and synergies.
Neural Network vs. Machine Learning
In the developing tech area, the terms neural networks vs. machine learning often surface. They signify two integral yet distinct components within the realm of artificial intelligence. Clarifying the nuances between these concepts is essential. It is vital for grasping their roles in shaping intelligent systems. Comprehending the distinctions between neural net vs. ML is critical. It provides a clearer perspective on their roles and apps in AI.
Defining Neural Networks
They are a pivotal subset within the broader area of AI. Neuronic net revolutionizes tasks that demand intricate pattern recognition and hierarchical learning. Here's a brief breakdown of their definition and purpose:
- Structural Inspiration. Drawing inspiration from interconnected neurons in the human brain.
- Hierarchical Processing. Facilitating hierarchical learning, enabling systems to comprehend intricate patterns.
- Deep Learning Subset. Neural Nets, especially in DL, involve multiple layers for advanced feature extraction.
- Pattern Recognition Mastery. Specializing in intricate pattern recognition tasks like image and speech recognition.
- Adaptive Learning. Exhibiting adaptive learning, refining their understanding of data over time.
Knowing the essence of neural networks vs. machine learning is crucial. They highlight their specialized role in mimicking cognitive functions.
Machine Learning Explained
It is a reformative subclass that empowers systems to learn from data. Also, it enables them to adapt and improve without explicit programming. Here's a brief exploration of its definition and app areas:
- Definition. Involves algorithms allowing systems to learn from data and improve without explicit programming.
- Adaptive Algorithms. ML algorithms adapt and enhance performance based on learned patterns.
- Application Areas:
- Predictive Analytics.
- NLP.
- Image and Speech Recognition.
- Recommendation Systems.
- Healthcare Diagnostics.
- Fraud Detection.
- Versatility. Adaptable to various tasks, making it a cornerstone in intelligent system development.
Understanding this subclass unveils its broad utility across domains. ML makes it a cornerstone in developing intelligent systems.
Comparative Analysis
Comprehending the differences and relationships between these subsets is paramount. Here's a concise analysis:
- Definition:
- ML: Encompasses a broader concept involving algorithms for systems to learn from data.
- Neuronic Networks: A specialized subset within ML inspired by the human brain's structure.
- Scope of Application:
- ML: Versatile, encompassing various learning approaches.
- Neural Net: Specialized in deep hierarchical learning for intricate pattern recognition.
- Learning Mechanism:
- ML: Utilizes various methods, including supervised and unsupervised learning.
- Neural Net: Involves interconnected layers for hierarchical learning.
- Pattern Recognition:
- ML: Encompasses a broader range of tasks.
- Neuronic Networks: Excel in complex pattern recognition tasks.
- Adaptive Learning:
- ML: Adaptive algorithms improve performance over time.
- Neural Net: Exhibit adaptive learning, particularly adept at handling diverse datasets.
Understanding these nuances clarifies how these techs coexist and contribute to the intricate area of AI.
Machine Learning vs. Neural Networks
In the dynamic area of AI, the distinctions between machine learning and neural networks play a crucial role. ML, a broader concept, employs diverse algorithms for learning from data. In contrast, neural networks, a subset of ML, mimics the human brain's structure for hierarchical learning. Distinguishing between these terms is essential for grasping their roles in intelligent systems. ML encompasses various approaches, while neural net emphasizes deep, structured learning.
The Role of Neural Networks in Machine Learning
Comprehending the symbiotic relationship between these subgroups is crucial. It unveils the nuanced ways neural nets contribute to the broader field. Here's a breakdown of their pivotal role:
- Hierarchical Learning Structure. Neural networks introduce a hierarchical framework, enabling ML systems to comprehend complex patterns.
- Pattern Recognition Mastery. Specializing in intricate pattern recognition, neural net elevates ML capabilities in tasks. Among them are image and speech recognition.
- DL Advancements. Neural nets employ many layers for advanced feature extraction, particularly in deep learning.
- Adaptive Learning Mechanism. Adaptive learning in NN contributes to ML's flexibility in handling diverse datasets.
- Complex Problem Solving. Neural net within ML tackles complex problems, making sense of intricate data patterns.
- Enhanced Decision-Making. NN's hierarchical learning and pattern recognition capabilities are helpful. They improve decision-making processes in ML systems.
Knowing how this concept plays a vital role in ML underscores their significance. It is in navigating and making sense of intricate datasets.
Distinguishing Features
Comprehending the nuances that set apart machine learning and neural networks is crucial. It is vital in unraveling the complexity of artificial intelligence. The key features differentiate these two subsets:
- Machine learning. Employs algorithms for learning and adapting from data, enhancing performance without explicit programming.
- Deep Learning. A subset of ML utilizing neural networks with multiple layers for intricate pattern recognition.
- Natural Language Processing. It enables machines to comprehend, interpret, and generate human-like text, enhancing language interactions.
- Robotics. Integrates AI for autonomous decision-making in physical tasks, advancing automation.
- Artificial Narrow Intelligence. Specialized in performing specific tasks, lacking general human-like intelligence.
- Artificial General Intelligence. Possesses broad cognitive abilities, showcasing intelligence across diverse domains.
Understanding these distinguishing features is essential. It sheds light on the roles and apps of ML and NN in the dynamic area of knowledge engineering.
Neural Networks vs. AI
The distinction between these subgroups is crucial. AI stands as the overarching term encompassing diverse technologies replicating human intelligence. The neural net, in contrast, represents a specialized subset within MI. They emphasize hierarchical learning inspired by the human brain. AI encompasses a broader spectrum of technologies. Neuronic networks have unique structures and functionality. They play a distinctive role in advancing the capabilities of intelligent systems. Understanding this relationship is essential for navigating the intricate terrain of AI hi-techs.
Positioning Neural Networks within AI
Exploring the intricate realm of AI is crucial. It involves understanding the nuanced role of neural networks vs. AI. Grub into the breakdown below to grasp how neural networks function within the expansive area of MI:
- Subset Specialization. Neural Nets function as a specialized subset within the broader field of AI. It emphasizes hierarchical learning mechanisms inspired by the human brain.
- Deep Learning Catalyst. Playing a pivotal in deep learning, NN uses many layers to ease intricate pattern recognition. It catalyzes advanced machine learning capabilities.
- Pattern Recognition Proficiency. NN excels in handling complex pattern recognition tasks. They significantly contribute to AI's skills in image and speech recognition.
- Adaptive Learning Dynamics. Within the AI framework, neural nets showcase adaptive learning mechanisms. They continuously refine their understanding of data over time for improved cognitive capabilities.
- Versatility Across Domains. Exhibiting remarkable versatility, NN contributes significantly to various domains within AI. Their impact is evident in NLP, image analysis, and the development of autonomous systems.
Knowing the symbiotic relationship between this concept and AI is imperative. It sheds light on their integral role in orchestrating intelligent systems. Also, it pushes the boundaries of AI capabilities to new heights.
H3: How Neural Networks Contribute to AI
They are pivotal in advancing AI. It brings about reformative contributions that enhance the capabilities of intelligent systems. Here's a detailed exploration of their significant impact:
- Hierarchical Learning. Computer-aided learning introduces hierarchical structures, enabling AI systems to comprehend complex patterns.
- Pattern Recognition Mastery. NN specializes in intricate pattern recognition tasks. It enriches AI's image and speech recognition capabilities.
- Adaptive Learning Mechanism. Adaptive learning in neural networks contributes to AI's flexibility. It allows systems to refine their understanding over time.
- Complex Problem Solving. Neural nets within AI are adept at tackling complex problems and making sense of intricate data patterns.
- Enhanced Decision-Making. Neural net's hierarchical learning and pattern recognition capabilities help improve decision-making processes.
- Versatility Across Domains. Computer-aided learning showcases versatility. They contribute to domains such as image analysis, NLP, and autonomous systems.
- Continuous Improvement. NN enables AI systems to improve continuously. They are adapting to new data and refining their performance over time.
Understanding the intricate contributions of neural networks vs. AI is vital. It highlights their collaborative role in shaping the area of intelligent systems.
Deep Learning vs. Neural Networks
The distinction between these hi-techs lies in the depth of their structures. Neural net refers to interconnected layers of nodes for hierarchical learning. Deep learning involves explicitly neural networks with multiple layers. The critical difference lies in the depth of these structures. Deep learning goes beyond traditional computer-aided learning. It employs more layers for advanced feature extraction and intricate pattern recognition. Comprehending this difference between deep learning and neural networks is essential. There both concepts play integral roles in shaping intelligent systems.
Deep Learning Defined
It is a subset within the broader ML and neural nets domain. They are famous for their complex architecture and hierarchical learning. Here's a comprehensive breakdown of its definition and significance:
- Neuronic Network Architecture. Utilizes bind layers of nodes for processing information hierarchically.
- Deep Neural Nets. Involves nets with multiple layers, allowing for advanced feature extraction.
- Pattern Recognition. Excels in complex pattern recognition tasks, such as image and speech recognition.
- Hierarchical Learning. Emphasizes learning hierarchical representations from data.
- Versatility. Applicable across various domains, from image analysis to NLP.
- Computational Requirements. It often demands substantial computational resources due to the depth of nets.
Knowing the intricacies of this subset reveals its profound impact on AI. It shapes the capabilities of intelligent systems.
Understanding the Relationship
Comprehending the differences between deep learning and neural networks is essential. Here's a detailed breakdown:
- AI as the Umbrella Term. It encompasses all technologies that mimic human intelligence.
- Machine Learning as a Subset. Utilizes algorithms for learning and adapting from data, falling under the broader AI spectrum.
- Deep Learning Within ML. A specialized technique employing neural networks with multiple layers for intricate pattern recognition.
- Neural Networks as a Component. A subset within ML mimicking the hierarchical learning structure of the human brain.
These nuances clarify how such concepts coexist and contribute to the diverse AI area.
Conclusion
Unraveling the intricacies of such subgroups is crucial. It provides a nuanced understanding of their roles in shaping modern hi-tech. AI is the umbrella, with ML as a versatile subset and deep learning as a specialized neural net technique. The NN, inspired by the human mind, contributes to hierarchical learning. Recognizing the dynamic interplay between these concepts is vital. It is pivotal for navigating the developing area of intelligent systems. This comparative analysis fosters an appreciation for the diversity and synergy within AI.